TECHNOLOGY EXECUTIVE UPDATE VOL. 1, NO. 1
AI-based systems work on vast amounts of structured and unstructured data to identify patterns and trends within that data. The ensuing analytics generates insights for decision makers. The systems, however, do not and cannot provide explanations as to why a certain recommendation is made.
Users want to move away from this black-box model due to lack of explainability. In fact, all AI systems stakeholders, including developers and vendors of AI solutions, want visible fairness and accuracy that would increase trust and use of these systems.
The underlying philosophy of AI-based systems is that they work on correlations, not causations. In other words, an AI-system can analyze millions or billions of records to correlate them and, thereby, arrive at the probability of a certain occurrence. The system can then present that probability in a way humans can understand and incorporate in their decision-making process. The system, however, cannot provide the cause for the probability of that occurrence. AI systems do not reason or argue, but simply execute the algorithms on the data provided.
Neural networks, in particular, work on correlating a vast amount of data that is used to train and validate the system and test its logic. Since AI systems work on probabilities, the better the data, the better the analytics. The algorithms do not provide any “reasoning”; therefore, the recommendations and subsequent decisions lack a human touch. Consequently, when a highly complex algorithm is executed on a large data set, chances of data bias and potential inaccuracies in the algorithm cannot be ruled out. Decisions based on poor or biased data or algorithms can lead to many social and business challenges, including legal wrangling and court suits. Legal challenges arising out of such unexplainable analytics have serious business and social repercussions.
Enter XAI
XAI is the discipline of going deeper within the AI system, identifying the reasoning behind the recommendations, verifying the data, and making the algorithms and the results transparent. XAI attempts to make the analytical recommendations of a system understandable and justifiable — as much as possible. Such explainability reduces biases in AI-based decisions, supports legal compliance, and promotes ethical decisions.
XAI is vital for the acceptance of AI and machine learning (ML) technologies in business and society. “Explainability” of an AI-based system includes but is not limited to:
-
The need to understand the context in which decisions are made based on the system’s recommendations
-
The need to justify the recommendations suggested by the system
-
The need to avoid biases in decision making
-
The ability to provide evidences in a court of law, if required
-
The ability to modify or override the recommended decisions should the context change
-
The ability to ensure that the recommendations are ethical and moral
What Are the Risks of Lack of Explainability in AI Systems?
Decisions based on AI systems impact individuals and societies every day. Systems analyze data in a wide variety of ways, ranging from pattern descriptions and predicting a trend to providing prescriptive analytics. Business decision makers rely on these recommendations in tactical, operational, and strategic situations. And, increasingly, automated systems (e.g., autonomous driving and robotic warehouses) execute decisions as well.
The analytics are based on the systems designed and coded by the developers and owned and executed by users. The algorithms are coded to traverse large data sets and establish correlations. But there is no onus on the AI system to explain its analytics and recommendations. An understanding of the data features and the high-level system architecture is not enough to explain or justify a particular recommendation. Biased data and algorithms lead to loss of trust and confidence in the systems and, worse still, moral and legal challenges. Biased decisions can ruin individual lives and threaten communities.
These risks are becoming increasingly apparent in AI-based decision making. AI systems lack contextualization, which presents interesting challenges. As Professor John H. Hull explains, “Teaching machines to use data to learn and behave intelligently raises a number of difficult issues for society.” For example, while the data that is fed into the system is factual, biases in the data can lead to biases in recommendations. Feedback loops in AI decisions can exacerbate the original biases, and biases in algorithms create further challenges that are difficult to detect before multiple system executions.
AI systems grow and expand their knowledgebase in an iterative and incremental manner, using large, historical data sets (also called big data) for analytics. Each decision based on an AI recommendation is fed back into the system. Additionally, data is used to train the system to make recommendations and also to test validity of the results. Incorrect, incomplete, or skewed data at any point can lead to skewed decisions. As fellow Cutter Expert Curt Hall states in a Cutter Advisor:
Because much of this data is historical, there is the risk that the AI models could learn existing prejudices pertaining to gender, race, age, sexual orientation, and other biases. Other issues with training and testing data can also contribute to bias and inaccuracies in ML algorithm development, particularly the use of incomplete data sets that are not fully representative of the domain or problem the developers seek to model.
AI systems also make rapid, split-second decisions that are not humanly possible. Humans cannot match the speed of crunching and correlating vast amounts of data. However, AI-based recommendations without explainability present substantial risks and challenges. Some examples include:
-
AI systems can help with medical diagnostics such as identifying the tiniest dot on a scan as the beginning of cancer by correlating millions of data points within seconds, but cannot explain why that dot is likely to become cancer.
-
AI-based systems have plotted COVID-19 pandemic pathways with reasonable accuracy, thereby helping the health domain to prepare for ICU capacities and vaccine administration, but cannot provide reasons for increased requirements, leaving that insight up to human scientists.
-
The systems can assist police with identifying the likelihood of a crime spot, but cannot provide the reason behind the increased activities.
-
In education, AI systems can predict which cross-sections of students are most likely to drop a course, but without reporting why.
Each scenario (and many more) has associated risks. Decisions based on these recommendations present ethical and moral challenges that are not within the scope of AI-based systems because they lack an understanding of the context in which the decisions are made. Therefore, despite the obvious advantages of speed and increasing accuracy, the vastness of data and the complexity of its analytics lead to situations wherein the “reasoning” behind those insights remains elusive.
Automation leads to straightforward predictions based on a clean set of data. For example, algorithms for autonomous driving, robotics processes in warehouses, or executing a stock market order wherein the context has not changed and there are no exceptions, can be coded relatively easily. Automated processes relieve the “routine” decision making by humans and free up that time for more creative utilization. However, as soon as the context changes and the nonroutine comes into picture, coding and execution become challenging. The uncertainty of the context in which the decisions are made presents a risk. Fully automated decisions that leave humans completely out of the loop are risky, especially if the context keeps changing. Users also have subjective interpretation of their needs, and their values keep evolving.
An explanation for an AI decision that is understandable to people is imperative for the acceptance of these systems in business and society. XAI is based on the need to provide a reason or justification for the analytics generated. The greater the adoption of AI in daily lives, the greater is the responsibility of the systems to explain the reasons behind the recommendations. In addition, analytical insights generated from AI should not violate the legal and ethical contexts of the regions in which they are executed.
Making AI Systems Explainable
AI models are only as good as the data fed to them and the algorithms that are coded within. Biases in models can crop up due to data bias, and biases in algorithms can result from a developer’s viewpoints. Natural intelligence (NI) provides a countermeasure to these biases, challenging the data and algorithm biases with its understanding of the context in which decisions are made. NI is the investigation of the underlying causes of decisions and the superimposition of values on AI recommendations. The intuition, experience, expertise, and associated knowledge of NI help alleviate the impact of biases in AI-based decision making.
Thus, one way forward in providing explanations in AI systems is to complement their recommendations with NI. While it is not possible to complement every decision, the system itself can be made to flag a small percentage of decisions to require NI before the decision is executed. Ajay Agrawal et al., recommend examining the results from the analytics in various contexts. They recommend examining qualities; for example: “Do men get different results than women? Do Hispanics get different results than others? What about the elderly or the disabled? Do these different results limit their opportunities?” Incorporating NI in decision making can be judiciously institutionalized in order to ameliorate the impact of out-of-context decisions. Biological neural network models have also been discussed as methods to help understand adaptive intelligence, combining AI with NI.
Providing explanations of the recommendations AI-based systems use to identify, define, and monitor decision making is an approach to mitigating biases. But alleviation of biases is not limited to testing the data. AI system developers must start their development work with a commitment to remain unbiased — in the use of data, coding the algorithms, and applying the results in decision making. Awareness of potential biases in each stage of development, testing, deployment, and usage can reduce negative impacts on decisions.
Each of the aforementioned stages of developing an XAI system benefits from the use of an Agile approach, which can help reduce the amounts of biases creeping into a system. Agile — with its iterations and increments — is ideally suited to reduce development bias because Agile iterations and increments make the entire development visible and transparent. Potential biases can be identified during the continuous testing within a sprint. Even though Agile is not documentation-centric, the opportunity to test continuously and to develop the solutions in a transparent way enhances explainability.
Detecting biases during deployment and operation of a system is equally important, although more difficult than detecting them during development. Once the AI system is deployed, the vastness of data and the multitude of data sources make the explanation of decisions even more challenging. The speed and accuracy with which biases in data and algorithms are resolved is also important in developing trust in the AI-based systems.
In addition to AI systems’ use of large amounts of data, variety in the data and the use of multiple data sources can help in alleviating potential biases. For example, a health AI system can use pandemic data from two different sources and undertake a comparison of the analytical results using the same algorithm. If the results are different beyond a set parameter, the data and the algorithms must be investigated further.
The contexts within which decisions are taken can also be recorded to the extent possible by the system. Opportunities for optimized business decision making can change based on the context, but usually the context is not stored (encoded in the system). Recommendation engines with rapid feedback loops based on the situation in which a decision is taken and whether that decision turned out to be of value provide additional capabilities to provide explanations and justifications.
Explainability of AI systems assumes an even greater focus when these systems do more than automate existing tasks. Optimization, more than automation, is where businesses derive value from AI, but these are precisely the situations where superimposition of NI is required to improve explainability. The optimization of business processes should follow the principles of iterative and incremental development based around agility that can improve the explainability of the algorithms.
AI systems also “learn” during execution. For example, an AI system learns from the experience of interacting with a customer and stores that information. Later, the stored interaction is used to enhance interactions with the next customer. ML algorithms continue incrementally to learn to handle a business problem and refine the answers. This iterative and incremental learning can reach extremely deep levels, leading to deep learning (DL).
Many consider DL to be resource hungry, unexplainable, and easily breakable. It requires huge training data sets, is unexplainable due to the depth of its multiple layers, and is fragile due to a lack of context in which decisions are being made. For example, a robot can learn to pick up a bottle, but if it has to pick up a cup, it starts from scratch. In DL, the logic behind the learning becomes so deep that it becomes impossible for the human mind to understand the reasoning and/or the algorithms behind the decision. Recommendations become unexplainable. In such situations, superimposing AI with NI reduces the speed of decision making but does not sacrifice the accuracy of those decisions. In fact, NI adds significant value to AI-based decisions by understanding the context in which decisions are made. Should the entire context, or scenario, in which a decision is made be “codable,” then those decisions can be included as input in the subsequent iterations of the AI-based system.
Table 1 summarizes the challenges of AI explainability and possible approaches to ameliorate those challenges.
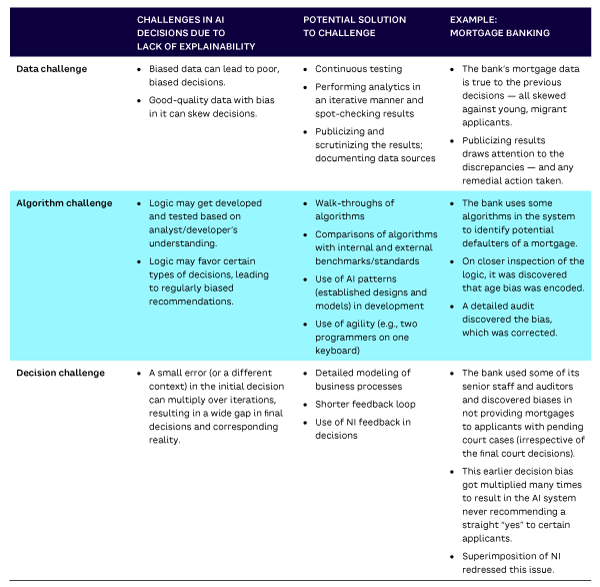
Conclusion
Due to their extremely complex nature, biases can creep in and multiply over time in AI systems. It is important to provide explanations of the recommendations the system makes. Certain industry-based initiatives, such as the Data & Trust Alliance, work toward alleviating the impact of biases in AI-based decision making, based on a framework that includes evaluation, education and assessment, scorecards, and implementation guidance.
This Update highlights the challenges of AI-based decision making in practice. We have explored some approaches to alleviating biases and providing greater explanations in decision making. Data, algorithms, and decisions themselves can lead to biases, and superimposing AI recommendations with NI can be an effective tool for handling those biases.
Acknowledgments
The author offers special thanks to his students, Julian Chauhan and Yash Jain, who reviewed this Update.