Experienced IT practitioners know that errors will occur. A big part of building and managing complex systems is dealing with risk management (which includes identification and mitigation strategies). This is hard enough when documentation and source code exist. But the current state of ML-based AI tends to result in opaque black boxes, which make this activity, um, challenging. David Biros, Madhav Sharma, and Jacob Biros explore the implications for organizations and their processes.
Article
Vulnerability and Risk Mitigation in AI and Machine Learning
Posted October 10, 2019 | Leadership | Technology | Amplify
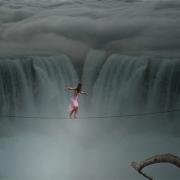
Don’t have a login?
Make one! It’s free and gives you access to all Cutter research.