CUTTER BUSINESS TECHNOLOGY JOURNAL VOL. 31, NO. 9
Pat O’Sullivan, an IT architect and Senior Technical Staff Member at IBM, starts from the premise that the principles of standardization and conformity that were developed for the data warehouse are equally applicable to a digital business to deliver a consistent view of information to many lines of business. He explores the characteristics of a system of common metadata that can define the links between an existing data warehouse and an emerging digital business, describing the components and characteristics of this new metadata layer and how it is essential to fueling the growth of the AI capabilities of a digital business.
Many of the principles that were developed for the data warehouse are just as relevant today when considering the evolution to a digital business. Specifically, the focus on standardization and conformity to deliver a consistent view of information to many lines of business (LOBs) has parallels when considering the need to ensure a consistent experience for customers and partners when using an organization’s different digital channels. Underpinning these principles is the implicit need for the active use and management of a coherent layer of metadata.
Using metadata to underpin various parts of the IT infrastructure is not new. The capability to build out from a single canonical reference framework for different IT systems, including data warehouses and the applications that feed them, has been a goal for many organizations for decades or, at least, a goal for the enterprise architects within them. Unfortunately, in the past we saw the balkanization of many IT organizations into separate and often totally independent entities to deliver such systems as the data warehouse, the separate operational systems, and the services layer. Developers of these systems often only paid lip service to whatever enterprise-wide framework of metadata and designs was in vogue. So what is different now?
This article explores the latest thinking on how to evolve a common metadata system that truly begins to provide a key integration point between the data warehouse/data lake and the growing set of digital processes. It explores the components and characteristics of this new metadata layer and how it is essential to fueling the growth of artificial intelligence (AI) capabilities across the enterprise.
The Data Warehouse’s Evolving Role in Digital Business
In discussing the broader digital business, it may help to delineate the various systems and applications that occupy the landscape of such a business. As shown in Figure 1,1 a high-level classification of such systems could be:
-
Systems of insight — data lake, data warehouse, and other associated analytics/business intelligence applications
-
Systems of record — ERP and other back-office transactional systems
-
Systems of engagement — Web portal, chatbots, and other applications used to engage with clients and business partners
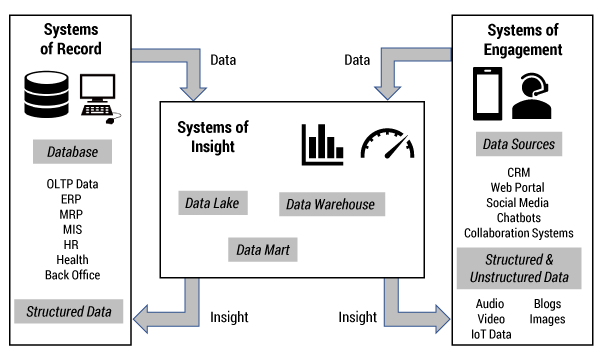
(Adapted from: Chen et al.)
The data warehouse was a response to the need to integrate and standardize key data and associated metrics from across a growing and divergent range of upstream systems that run the business — the systems of record. Such a one-stop shop of curated, managed, classified, and cleansed data provided the perfect basis for the creation of a multitude of analytics and reports to support many internal and external business needs, from various LOB reports to executive dashboards to the provision of data to various regulatory bodies.
The delivery of these different business needs was felt to justify the often time-consuming and costly efforts to create and maintain data warehouses. In most cases, there was also a very clear delineation between the world of systems that ran the business — the systems of record and systems of engagement — and the world of the data warehouse and analytics — the systems of insight.
A significant evolution in recent years has been the blurring of traditional frontiers between the data warehouse (or data lake) and the different systems running the business. This is driven by the demand for embedded analytics in the digital business as well as the need for the data management systems to be more responsive in processing real-time or streaming data. One outcome was to dispense with the formal ETL layer that was the de-facto representation of the boundary between the data warehouse and these other systems.
Another change is the growing need for the delivery of automated capabilities across the enterprise, such as the expanding role of chatbots in the provision of a delivery channel; the birth of completely new business areas such as vehicle telematics in insurance; the availability of highly personalized experiences to customers based on their past purchasing history, expressed preferences, and current location; the increasing need for on-the-fly automatic analysis; and the need for classification of data as it enters the data warehouse or data lake.
Finally, one of the most significant common overall trends to emerge is the role of AI to revolutionize systems deployment across the technology landscape, driven by the need to deliver increased levels of automation and enhanced adaptability, as well as to address new use cases or business needs. The growth of such automated and cognitive systems drives the need for more expressive and adaptive forms of metadata to enable and underpin such AI, which, in turn, raises questions about the traditional role of such metadata components as the data catalog, the business vocabulary, and the data model.
The Role of Metadata and Modeling Activities
In the past, explicit schemas or models assisted IT and IT-savvy users when understanding, navigating, using, and extending the systems for which they were responsible. This approach applied to the various systems that ran the business as well as to data warehouses and other analytical systems. With this predominance of predefined schemas, the data model took on a major role in enabling organizations to make sure that the specification of such database schemas reflected, as far as possible, the business and associated IT objectives that underpinned and funded the development of the data warehouse.
However, the advent of big data technologies with an emphasis on the ease and speed of ingestion of large amounts of data into a data lake — as opposed to the often-complex traditional ETL processes for loading into a data warehouse — meant far less focus on defining schemas or structures. Many data sets being loaded into the data lake either have no schema or bring their own implicit schemas. With many early data lakes, this sacrifice of structure to enable fast and easy ingestion was acceptable because the focus was either experimental or was initially intended to supply data to data scientists in support of their predominantly discovery-related activities. However, as the data lake becomes more mainstream and required as part of an overall data management infrastructure serving analytics with improved data provenance, the focus now shifts toward how to achieve an adequate level of governance of such data lakes. This is where the data catalog provides a central canonical reference point of business meaning to underpin any data governance activities of the data lake.
Physical Conformance vs. Catalog Conformance
One way of thinking about this is as a shift from traditional physical conformance to the achievement of conformance via the catalog (see Figure 2). Essentially, one of the traditional objectives of the central warehouse is to achieve conformance of the data by ensuring it is physically stored, subject to standard schema specifications; in other words, to achieve physical conformance. With the data lake, such conformance of data to a standard schema is neither desirable nor possible in any economic way; indeed, many big data/data lake exponents would call out as an anti-pattern any attempt to enforce schema standardization on data stored in Hadoop or other NoSQL formats. So, if the physical conformance of the data structures is not possible, how can an organization achieve any degree of governance of the data lake? One option is to consider achieving conformance via catalog conformance.
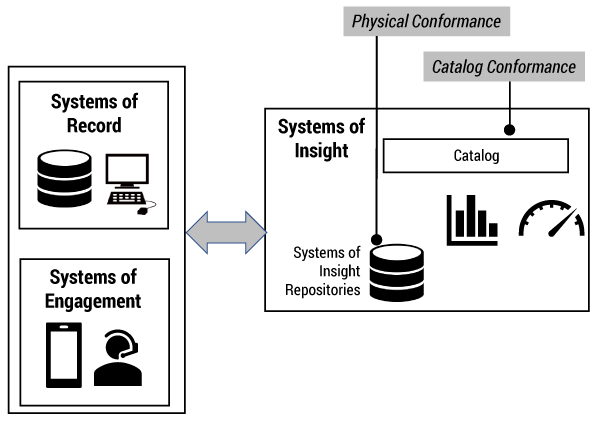
With catalog conformance, the objective is to ensure that the data within the data lake is mapped to an overarching catalog of interrelated business terms that effectively provide the business language used to describe the contents of the data lake. Thus, the contents of each data set within the data lake (or portion of it to be subject to data governance) are mapped to this catalog of business terms. Any users or applications accessing the data lake can use the catalog as a basis for determining the location, relationships, quality, and other characteristics of those data elements.
As organizations define and build this emerging business vocabulary for a very fluid and interrelated data lake, the question arises around the scope of such a business vocabulary — specifically, whether this catalog of terms is just concerned with the analytics area or whether it needs to have a wider scope, even to the extent of acting as the vocabulary for the whole enterprise.
A critical aspect of this new approach to the provisioning of metadata is the existence of a layer of business metadata separate from but integrated with the metadata describing the physical details of the underlying structures. In the past, data warehouse metadata was often so focused on the data’s physical aspects that it was of little use to people trying to build other non–data warehouse applications. However, the evolution of data management metadata solutions now includes a semantic layer more focused on business language and business constraints. This means that the potential opportunity arises to use this metadata layer across the broader digital business.
In considering a broader scope for any data lake business vocabulary, it is often necessary to decide whether the scope is to just represent the business language of the data lake/systems of insight or whether it extends also to represent the core business language of other systems across the enterprise. Such a decision fundamentally impacts how this business vocabulary is designed, what personas and activities it is assumed to support, and what technology should be used to underpin the catalog.
For example, one approach is to build out a more expressive semantic layer of metadata with more extensive ontologies rather than limiting the metadata to the more traditional taxonomies or glossaries typically found in data management metadata repositories. Such ontologies have a growing track record of practical use in underpinning the Semantic Web, as well as being viewed as key to the recent evolution of some cognitive systems. In this case, the focus shifts toward a metadata layer that is not just centered on the business meaning of the data elements and the type hierarchies needed for systems of insight, but is extended to cover the business rules, extended constraints, and relationships needed to reflect the additional needs of the systems of record and systems of engagement. Such an ontology may thus start to record the range of entities and intents needed to underpin the logic of chatbots, or the specific type hierarchies needed to support document discovery by customer agents.
In some cases, a pragmatic decision may be taken initially to limit the focus of any such business vocabulary to just the systems of insight. In other cases, the decision may be to define a cross-enterprise business vocabulary. In the latter case, a critical success factor of this broader use of metadata across the enterprise is the role of a chief data officer to own and champion the necessary cross-LOB cooperation, funding, and process of governance and change management.
Another alternative is a looser collaboration where organizations responsible for the different systems may use portions of this business vocabulary, but no significant effort is expended to enforce standardization across the enterprise. In fact, in many cases, this last approach becomes the default as the different individual developments progress in delivering point solutions across the systems of insight, record, and engagement.
The Future Potential Delivered by AI
Whether it is looking at the new function of metadata in the systems of insight or in supporting other areas of the emerging digital business, the increasing use of various AI capabilities is completely changing the role and usage of metadata. One common pattern is the shift from metadata being defined top-down to bottom-up. Specifically, there is an effort to move away from metadata being predominantly owned by information architects or enterprise architects to underpin mainly design-time activities with subsequent top-down deployments of metadata artifacts to the various runtime systems. Instead, there is a bottom-up demand for metadata to address specific areas, whether that is a vocabulary to govern a data lake; a type hierarchy used to assist natural language processing (NLP)–based extraction of key terms, obligations, and actions from unstructured data; or the need for entities and intents to be used in building the logic flow of a chatbot. In many such cases, the demand for metadata is coming from specific point projects within departments, as the relationship between central IT and the business changes, and as such departmental efforts gain greater levels of autonomy.
Another common pattern is the ever-increasing presence of AI applications and their growing demand for high-quality metadata. Examples include the need for an effective structured-type hierarchy to support the processing of any NLP and the need for extensive and rich metadata of the data lake to support any AI-based discovery activities.
A major question is determining how to achieve a coherent strategy for the use of metadata across the enterprise to underpin such AI-based activities. There are benefits to defining an integrated framework of metadata that spans all aspects of the digital business. Building some of the aspects of a metadata framework can be expensive and may require specialized skills (e.g., the creation of an ontology needed to support many of the AI applications), so it may be more economical to do so centrally for many parts of the business rather than doing so piecemeal. Also, a centralized or at least coordinated approach means more reuse and commonality of such metadata artifacts across the business, resulting in fewer potential inconsistencies. In some cases, such an integrated approach to the use of metadata across the full breadth of the digital business may not just be beneficial from a business efficiency perspective but necessary from a regulatory compliance perspective. Increasingly, regulators are demanding a holistic approach. For example, the regulation on risk data aggregation from the Basel Committee on Banking Supervision doesn’t just demand the provision of the necessary reporting data but insists on the demonstration of the provenance of such data right back to its source in the systems of record. Another example is the European Union’s General Data Protection Regulation (GDPR), which requires a holistic approach to the storage and protection of personal data across the systems of insight, systems of engagement, and systems of record.
Future Metadata Frameworks Offer Enhanced Digital Business Solutions
The increasing importance of a coherent organizational strategy to maximize the exploitation of data for growing a digital business is clear. There is a need to locate, consolidate, classify, and access the necessary data — often data of many different formats stored in different areas of the enterprise as well as external data — to drive many different aspects of a successful digital business. Examples include the increased use of AI-driven health monitors in hospitals to enable the early detection of combinations of potentially harmful symptoms; the ability of banks to offer more advanced wealth management applications that include access to far deeper research or provide a service to a wider range of potential customers; and the improvement in insurance of catastrophe risk via the use of machine learning models to automatically assess the severity of damages and predict repair costs of property based on historical data.
To achieve these types of capabilities in an efficient and scalable manner, the digital business needs to be able to identify, extract, transform, contextualize, and distribute the necessary data. The data warehouse or data lake is a critical part of this data ecosystem, and many different aspects of the digital business can exploit this resource in a consistent way by means of a shared metadata framework. Such a framework must be accessible and meaningful to the full range of business and technical users. It must be able to react to the inevitable changes that will occur in the business circumstances of the enterprise, such as changes in behavior of key customer demographics, the entrance of new competitors, and the impact of regulatory changes. This framework also must adapt to likely technological advances — not only the continued growth in the application of cognitive technologies but the potential advent of other applications in areas such as blockchain or the increased use of Linked Open Data to grow the knowledge base of the digital business.
While the need for expediency in achieving short-term goals is understandable and at times necessary, the adoption of such pragmatism is only scalable in the long term when allied with an evolving cross-enterprise metadata layer. This is especially true when one considers that change will not happen in just a single area but is likely to come from a range of disruptive events across a range of technical and business dimensions. Such a level of change places a further premium on the ability of the digital business to react in a holistic manner.
It may be beneficial to think of this metadata layer not as one single collection of terms, definitions, constraints, and rules, but more as a web of different but interrelated networks of knowledge: a network of business knowledge encapsulated in the systems of record, a network of the extensive data knowledge available in the systems of insight, a network of user knowledge from the systems of engagement, and perhaps a network of canonical knowledge to provide the central spine of metadata against which all other networks can be mapped and aligned (see Figure 3). This federation of different networks enables the growth of the necessary interoperability of knowledge across the digital business, including the data warehouse, but also provides the necessary degrees of freedom to the individual areas.
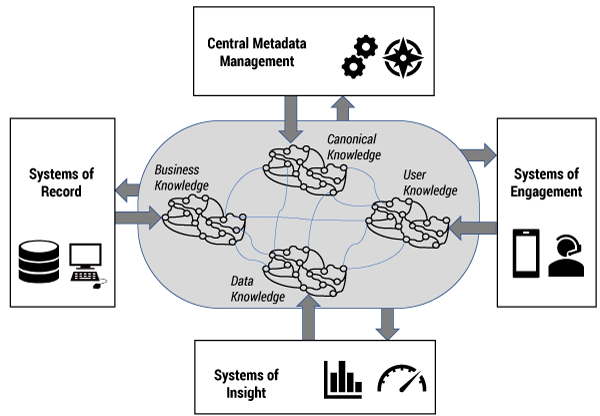
As the digital business continues to expand, such an integrated approach to the management of metadata across all its components is a logical extension to the traditional integrated approach to data first specified in the data warehouse 30 years ago. The benefits of the traditional data warehouse of conformance, consolidation, and consistency can now be extended with a more adaptable, scalable, tightly integrated, metadata-driven ecosystem to ensure that the digital business continues to receive the necessary fuel of data.
1 For a more detailed explanation of this classification of the different systems, see “Systems of Insight for Digital Transformation: Using IBM Operational Decision Manager Advanced and Predictive Analytics.”